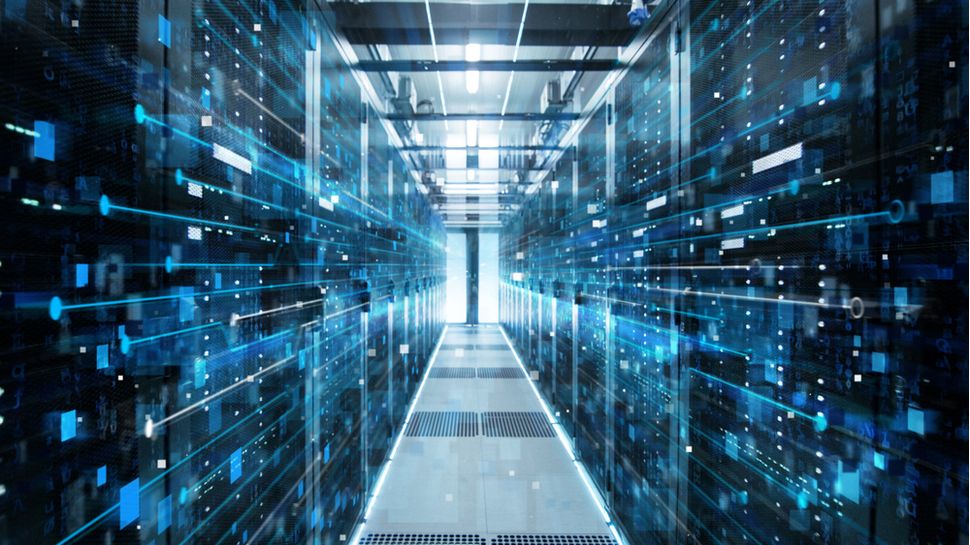
Three reasons we’re witnessing the death of the dashboard
Table of Contents What is a Dashboard? Reason #1: Meaningful insights into “why” require hours of
Table of Contents
In the last few years, many businesses have successfully transitioned from traditional business intelligence (BI) to self-service analytics. And overall, the change has had a significant impact.
Data analytics is more democratized. Insights are easier to understand. Even back-end processes like data preparation have been simplified.
However, with data growing in scale and complexity every day, and the demand for actionable insights getting louder and more urgent, BI dashboards are no longer up to the task.
What is a Dashboard?
Dashboards are a core component of self-service BI that allows users to visualize data and interact with elements according to a specific set of rules created by IT professionals and data analysts. Delivering insights through these visualizations, like charts and graphs, they answer users’ high-level questions about standard KPIs. Users can also filter and drill into views of data manually within dashboards to answer important follow-up questions.
If a question requires more information than an existing dashboard can offer – e.g. the focus shifts from quarterly sales numbers to a particular product’s sales in Kentucky over the last 30 days – new requests have to be sent to IT. These specialists then create new dashboards around the specific data sets users need to generate deeper data insights.
While dashboards make certain insights more accessible and useful for end-users, they have limits. In fact, dashboards can actually make it harder for business users to make data-driven decisions. Here’s why:
Reason #1: Meaningful insights into “why” require hours of manual analysis
To make intelligent business decisions, everyone (in every department and at every level) needs to know what the data says. They need to know what the high-level metrics are, and they must be able to dig into the reasons behind “why” metrics change and the differences between cohorts. And if businesses cannot get these deeper insights in a timely manner, they end up bridging insight gaps with assumptions and making gut decisions.
But since dashboards are only designed to deliver high-level answers automatically, discovering the “why” takes a lot of additional work. In fact, analysts can spend hundreds of hours per year, manually slicing and dicing data to coax out these deeper insights. And if the original dashboard can’t dig deep enough, new dashboards are needed.
Unfortunately, if data analysts take the time to do manual drill-downs or IT has to develop a new dashboard, organizations can miss big opportunities, first-mover advantages, and competitive business models. And smaller tasks, like marketing campaigns, customer support, and employee training can fall behind industry best practices – leading to a poor customer experience and inefficient operations.
In other words, it’s a lose-lose situation for everyone involved.
Reason 2: They don’t fit the modern workflow
Dashboards are designed to give simple, aggregated answers to user questions. As a result, businesses often need dozens, if not hundreds, of dashboards to answer the critical “why” question.
For example, sales teams might need a dashboard to see how Q3 sales stack up against last year’s numbers and compare the performance of individual states and regions. But if a sales lead spots a problem in Florida, they’ll need new dashboards to understand why. In this case, it might involve digging into relevant factors like demographics, popular sales channels, supply chain logistics, inventory management, and so on.
Unfortunately, every time a dashboard is added to the organization’s “library”, answers get harder to find. Analysts must open multiple dashboards and reports to see all relevant insights, simultaneously. And they have to run manual analysis on those insights to get a more comprehensive and valuable answer.
This is not a modern way of acquiring knowledge and looking up information. It’s downright old-school. When we look up information on our smartphones (or talk to a voice assistant like Alexa), the technology does the heavy lifting for us. The AI uses natural language processing (NLP) to understand the intent behind the questions, filters through trillions of results in milliseconds, and delivers useful answers in a way that makes sense to us (NLG).
Since dashboard and BI technology don’t have the capacity to do any of those things, users are stuck with manual analysis, interpreting data on their own, and pinpointing ad hoc insights they should act on immediately. And that not only opens the door to mistakes but takes lots of time.
Reason #3: The value of dashboards has become commoditized
Besides not being as widely used as they were originally intended (due to user frustration and lack of trust in the data), self-service BI tools aren’t delivering on their promise to help businesses make tough decisions. They simply don’t drill deep enough, fast enough to make a meaningful difference to users. Opportunities are long gone by the time they’re found to be a good move for the business, and often, revenue and customers are lost in the process.
Given the fact that machine learning analytics tools can generate deep insights in real-time, instead of hours or days after the initial query, investment into dashboards and reporting isn’t worth it for organizations who are truly aspiring to become insights-driven. There are better ways for data analysts and business users to attain actionable insights without relying heavily on data scientists.
What’s next for BI? Augmented analytics.
Augmented analytics addresses the limitations of dashboards in a few distinct ways. First, it automatically sources data from platforms, data lakes, and integrated tools and prepares it for analysis – a process that data scientists would ordinarily do by hand. It also allows users to type natural language queries into the search bar – like “How many shoes did we sell in Wisconsin last month?” – which triggers algorithms to automatically break down the data query to answer the question in both natural language (NLG) and visual formats.
Augmented analytics solutions will even show you the “why” behind the “what” and highlight predictive and prescriptive ideas to capitalize on with a few clicks. This means data analysts can step away from the monotony of building dashboards and unlock powerful, creative decision-making for all teams and departments.
In fact, using augmented analytics, improving productivity of the data analyst by 30 percent is easily within reason. And when applied to a business with 1,000 employees, where 50 work on data manually for 5 hours per week, that 30 percent timesaving can add up to 3,900 hours per year — nearly the annual workload of two full-time employees
As a result, businesses don’t just get better insights, but they also make better use of their employees’ time and skills.
Ajay Khanna, CEO, Tellius